Andrej Karpathy on AI infra of the future, making AI accessible
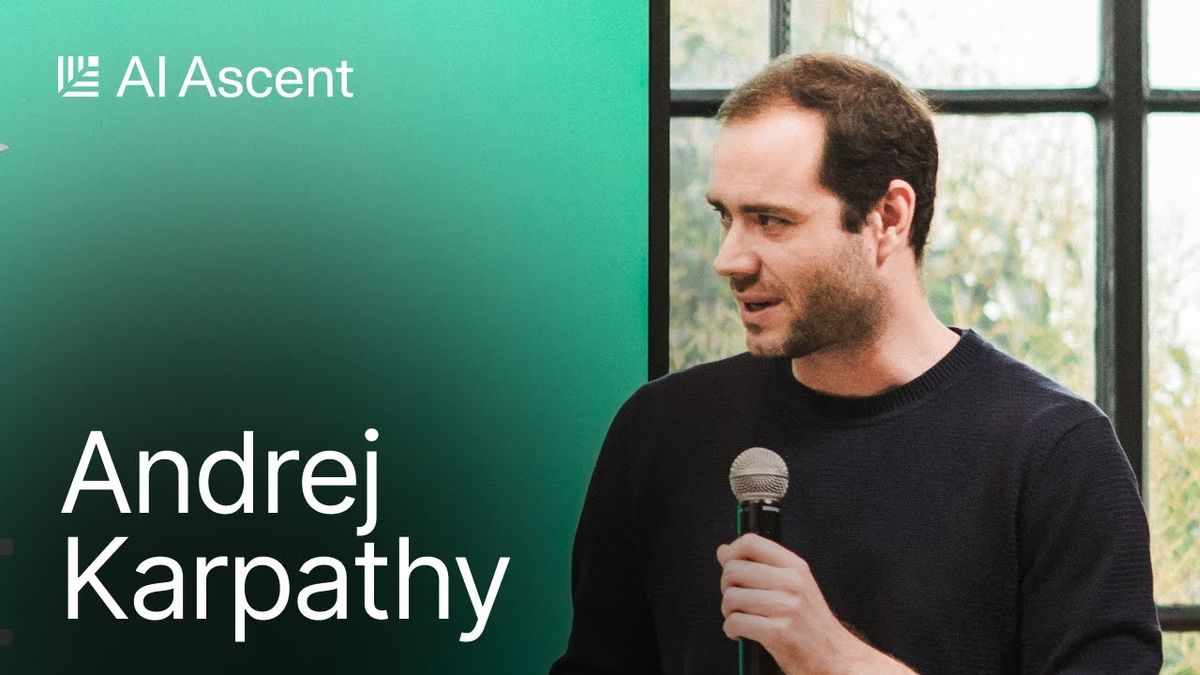
Transformer is kind of designed for the GPU…we want an architecture that is fundamentally extremely parallelizable. – Andrej Karpathy
In a conversation between Andrej Karpathy, a founding member of OpenAI and former Sr. Director of AI at Tesla, and Stephanie Zhan at Sequoia Capital’s AI Ascent, key insights into the future of the AI ecosystem, the importance of open-source models, and the need for innovation and ethical considerations in AI were discussed.
The dialogue also touched upon the leadership style of Elon Musk and the role of startups in the AI landscape.
Table of Contents
- Vision of a customizable AI OS
- AI infrastructure needs to adapt to the new data workflows
- Need for open-source models
- Importance of collaboration, innovation, and ethics in AI
- Enhancing AI model efficiency
- Democratizing access to AI
- Elon Musk’s leadership style
- Need for advancements in AI training methods
- Complexity of training AI models
- Strategies in model development
- Importance of educational resources and collaborative tools
- Revolution of the Transformer architecture
- Role of startups in the AI field
Vision of a customizable AI OS
The future of Artificial General Intelligence (AGI) is envisioned as a customizable ‘AI OS’ that integrates various modalities to create specialized agents for diverse tasks.
This shift towards AGI marks a significant advancement towards more attainable AI capabilities, paving the way for innovative applications and services tailored to specific needs.
AI infrastructure needs to adapt to the new data workflows
Andre believes that AI infrastructure needs to adapt to the new data workflows, pushing for better alignment between computer architecture and the demands of AI models.
– Scale will remain a primary determining factor, but expertise in infrastructure, algorithms, and data handling will also be crucial for AI infrastructure development.
– Precision reduction, sparsity, and improving the Von Neumann architecture of computers are potential areas for evolution to make AI infrastructure more efficient.
– Building a more energy-efficient and optimized AI infrastructure will be essential for the future of AI development.
There is a need for better ways of training models, where models learn to solve problems based on their own capabilities and psychology, rather than relying solely on human feedback.